Revenue teams aren’t ready for AI. Here’s why- and how you can prepare
Stay up-to-date with data-backed insights
Thank you for your submission.
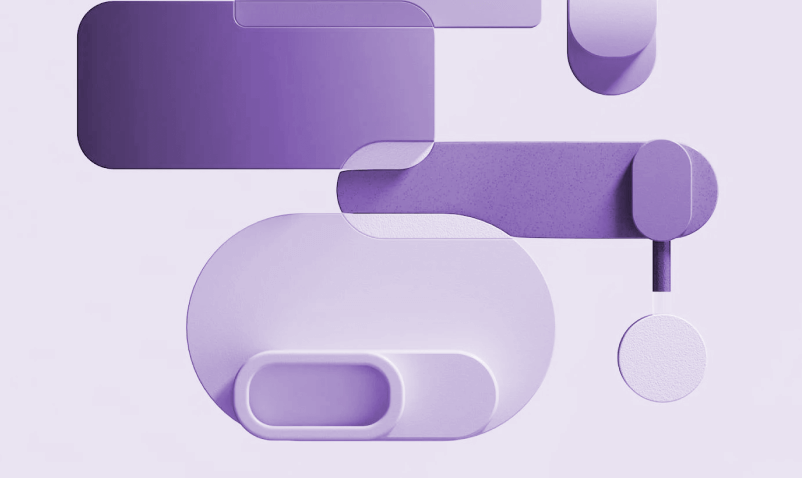
Imagine if your doctor gave you a diagnosis based on 1% of your medical information.
Imagine if your accountant gave you tax advice based on 1% of your financial information.
That’s exactly what revenue leaders are getting from their revenue technology—and what makes them so unprepared for AI.
A typical sales conversation has about 6,000 words, whereas a typical hand-typed call summary has 40–60 words. Even if that summary is directionally accurate, it omits 99% of the conversation.
This doesn’t just make it hard for leaders to get strategically valuable insights from customer interactions. It makes it impossible to realize the true value of AI. AI is no longer a glint in some technologist’s eye; it’s here, and the most prepared businesses are leveraging it for superior outcomes.
But the quality of AI-driven insights depends on one common element: data. Great data—comprehensive capture, solid organization—is the fuel AI needs to produce superior insights. If you’re looking at 1% of your data, your AI applications will be generating insights based on 1% of the picture—problematic when it comes to any AI use cases, from the simplest follow-up emails to the most high-level strategic decisions.
As CRO, I’ve long beat the drum of “Update the CRM.” But AI tools demand better. It’s time to march to a different tune.
The 3 facets of AI-driven revenue intelligence- and how data capture is core to each
1. Capture 100% of the data from customer and buyer interactions
A 1% summary of customer and buyer calls used to be the best we could hope for. That’s just not the case anymore. Tools (like Gong) exist today to capture 100% of these interactions, giving companies the richest possible store of data from which to cull insights.
I can’t stress enough that data capture is the foundation of getting value from AI-based tools. If an AI-based tool only knows the seller’s summary—“Great demo, likely to win”—it can’t write a targeted follow-up email. But if it has every word of the call, and the domain expertise to put it in context, it can turn a 6,000-word transcript into an accurate shortlist of key takeaways.
First things first: Capture everything. Turn every word into raw material for analysis.
2. Interpret/understand that data
The next layer after capture is interpretation—not just what the buyer/customer says, but what they mean.
Take, for example, the moment during a call when a buyer mentions a competitor. If they mention the competitor early in the call, that likely means they’re an informed buyer—they’ve done research on the space and have some sense of the different players. If they mention them later in the call, it’s often in the context of asking for a discount.
There are such moments that an AI system that’s been trained on billions of global data points will be able to recognize. But it can only deliver these insights if it’s looking at the entire transcript of a conversation, not just a summary.
3. Drive action based on that data
When you analyze vast stores of data, you ultimately look for coordinates for superior decision-making. This is both at the micro-level—within specific deals—and at the macro-level: impactful changes to your business’s overall strategic direction.
Today’s AI tools have the power to understand your OKRs and track them against seller behavior—the words they use, the percentage of time they talk during sales calls, the customer profiles they target, and more. This is the Holy Grail of revenue intelligence, and it’s only possible with a foundation of exquisite data capture.
If you’re not capturing 100% of your customer interactions, you’re looking through a peephole while your competitors get the full field of view. The longer you take to solve your data capture problem, the worse it will get—and the farther your competition will pull ahead.